
SES & Stats Dissertation Defense
February 13, 2025 @ 1:00 pm - 3:00 pm
Sirui Li (IDSS)
45-600B
Event Navigation
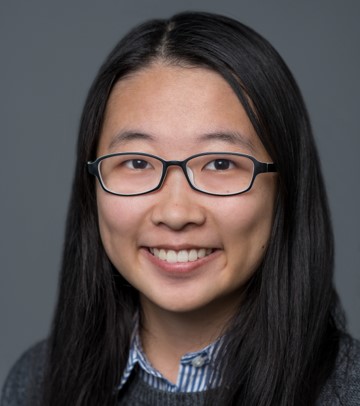
Learning-Guided Optimization for Intelligent Mobility Systems
ABSTRACT
Efficient and reliable mobility systems are essential to modern-day society, with broad impacts ranging from day-to-day commuting, public transportation, emergency response to last-mile package delivery and freight logistics. Autonomous vehicles have the potential to improve mobility efficiency and convenience but also raise questions about reliability and feasibility of deployment. The first contribution of this thesis is a set of novel, principled control-theoretical analyses that provide strong stability and reliability guarantees for autonomous vehicles and human-compatible driving, and they further covers emergent traffic behaviors in mixed-autonomy systems. While these theoretical guarantees offer valuable insights, mobility systems are inherently complex, and their overall performance often relies on solving difficult optimization problems, many of which are combinatorial, thus presenting significant scalability challenges. Overcoming these challenges requires innovative approaches that extend beyond traditional control techniques. This thesis further contributes a set of machine learning-guided optimization algorithms that significantly enhance the efficiency and scalability of solving combinatorial optimization problems. These algorithms have proven effective across a wide range of mobility-related applications. Compared to state-of-the-art solvers, they achieve 10× to 100× speed-up in large-scale vehicle routing problems, 35% to 70% solve-time improvement in various mixed-integer linear programming problems, and up to 54% acceleration in long-horizon scheduling problems. These advancements open new possibilities for efficient decision-making in large-scale transportation systems, enabling smarter, faster, and more adaptive mobility solutions. Combining learning, optimization, and control, this thesis demonstrates the potential of learning-guided optimization and principled control-theoretical analysis to address the increasing complexity of modern mobility systems.
COMMITTEE
Cathy Wu (chair & advisor), Alexandre Jacquillat, David Simchi-Levi
BIO
Sirui Li is a final year PhD student in Social and Engineering Systems (SES) and Statistics (IDPS), advised by Prof. Cathy Wu. She is a recipient of the Amazon Robotics Fellowship and the Michael Hammer Fellowship. Prior to MIT, She received her bachelor degree in Computer Science and Mathematics from Washington University in St. Louis. Her research interests broadly lie in the intersection between machine learning, operations research, and transportation. She has developed learning-guided algorithms to speed up combinatorial optimization solvers for efficient large-scale optimization. She is also interested in control theory analysis and reinforcement learning for mixed-autonomy transportation systems.
EVENT INFORMATION
Hybrid event. To attend virtually, please contact the IDSS Academic Office (idss_academic_office@mit.edu) for connection information.